.png)
Uncover Healthcare Fraud, Waste and Abuse With Speed and Accuracy
Detect and prevent improper payments with a focus on high-value cases and maximum ROI
Fraudsters don’t play by the rules. Your detection strategy shouldn’t either.
Shift’s healthcare-trained AI models go beyond static, rules-based detection methods to increase detection accuracy, uncover higher-value healthcare fraud, waste and abuse, and boost the efficiency of investigators.
Investigators should spend their time investigating. They shouldn’t waste time combing through sources for the right data or information. With enhanced plan data, combined with extensive external data, Shift gets the right insights to investigators faster.
Investigative detail all in one place
Uncover related providers
Alerts with context and action
Any data, any format
Healthcare fraud, waste and abuse tactics are constantly evolving, and investigators need the right tools to keep up. Shift’s powerful AI goes beyond rules-based methods, uncovering higher value FWA and sharpening accuracy with continuous learning.
Predictive variables
Entity resolution
Continuous learning
Natural language processing
Shift’s automated relationship and network analysis helps investigators identify related entities, suspected kickbacks, collusion and tackle high-value complex schemes.
Simplify complex fraud
Related entities
Network analysis
Related alerts
Boost team productivity and collaboration, leverage automation opportunities and organize complex casework with integrated case management features.
Assign & triage cases
Streamline communication
Activity tracking
Stay organized, on track and well prepared for any case handoffs or audits with step-by-step investigative activity tracking.
KPIs & reporting
Powerful detection across the full Payment Integrity lifecycle
Shift breaks out of the confines of static, rules-based detection methods with continuous learning, evolving schemes - capturing emerging FWA and high-value cases while automatically providing investigators with the details and data they need to accelerate post-pay investigations.
Good case management begins before the case even starts. Shift gives users informed alert assignment and triage capabilities, knowing what investigative workloads look across a team. Easily collaborate, communicate and request documents all without leaving the platform. Handing off a case or preparing for an audit? Shift's activity tracking keeps all the action in one place for clear, concise documentation.
TIME TO SHIFT AWAY FROM THE TRADITIONAL FWA DETECTION APPROACH
Healthcare fraud, waste and abuse is constantly changing - is the traditional approach enough? Dive into the traditional approaches to fraud, waste and abuse detection using rules-based tactics and the increasing shortfalls in relation to evolving fraud and demands from health plans. Learn how an AI-driven approach to improper payment detection can upend traditional approaches, with actionable insights and increased collaboration across the plan.
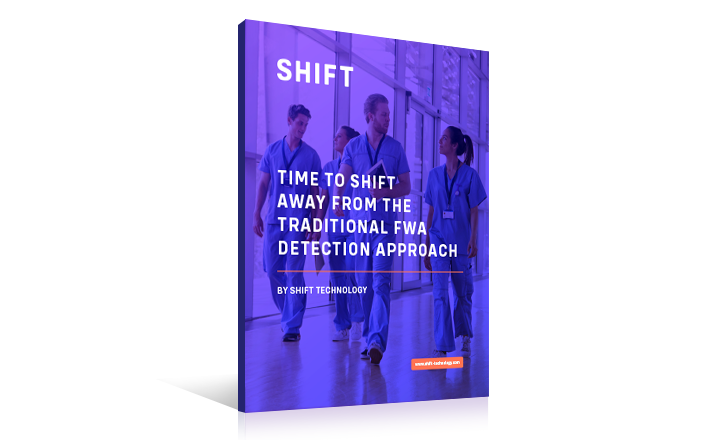
Industry Resources
.png)
.png)
.png)

Learn how Shift can help you successfully identify, investigate and prevent more fraud